Table Of Content
When an experiment includes multiple dependent variables, there is again a possibility of carryover effects. For example, it is possible that measuring participants’ moods before measuring their perceived health could affect their perceived health or that measuring their perceived health before their moods could affect their moods. So the order in which multiple dependent variables are measured becomes an issue. One approach is to measure them in the same order for all participants—usually with the most important one first so that it cannot be affected by measuring the others. Another approach is to counterbalance, or systematically vary, the order in which the dependent variables are measured. One sort of complexity mentioned was multilevel designs, but there are many complexities that can enter designs.
2.3. Assigning Participants to Conditions¶
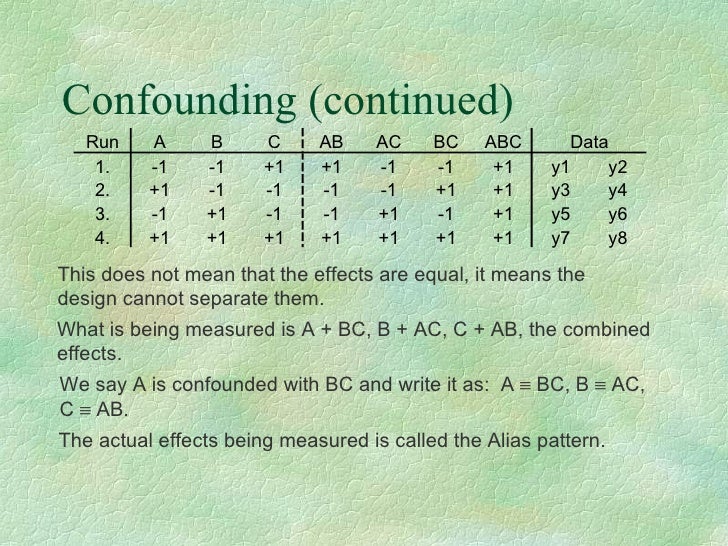
Instead, the experimenter changes the levels of the independent variable and then observes possible changes in the measures. This kind of design has a special property that makes it a factorial design. That is, the levels of each independent variable are each manipulated across the levels of the other indpendent variable. In other words, we manipulate whether switch #1 is up or down when switch #2 is up, and when switch numebr #2 is down.
Design of lipid-polymer hybrid nanoparticles for therapy of BPH: Part I. Formulation optimization using a design of ... - ScienceDirect.com
Design of lipid-polymer hybrid nanoparticles for therapy of BPH: Part I. Formulation optimization using a design of ....
Posted: Sun, 23 Feb 2020 07:34:51 GMT [source]
3. Factorial designs: Round 2¶
Ultrasonic pulse velocity and physical properties of hybrid composites: A statistical approach - ScienceDirect.com
Ultrasonic pulse velocity and physical properties of hybrid composites: A statistical approach.
Posted: Wed, 25 Jan 2023 15:58:13 GMT [source]
In other words, the effect of wearing a shoe does not depend on wearing a hat. More formally, this means that the shoe and hat independent variables do not interact. It would mean that the effect of wearing a shoe on height would depend on wearing a hat. But in some other imaginary universe, it could mean, for example, that wearing a shoe adds 1 to your height when you do not wear a hat, but adds more than 1 inch (or less than 1 inch) when you do wear a hat. This thought experiment will be our entry point into discussing interactions. A take-home message before we begin is that some independent variables (like shoes and hats) do not interact; however, there are many other independent variables that do.
3 - The Two Factor Mixed Models
Many of our design dimensions ultimately refer to different ways in which the qualitative and quantitative research components are interdependent. Different purposes of mixing ultimately differ in the way one component relates to, and depends upon, the other component. For example, these purposes include dependencies, such as “x illustrates y” and “x explains y”.
(e) Confirm and discover – this entails using qualitative data to generate hypotheses and using quantitative research to test them within a single project. The ezANOVA() function provides us with more detail, for instanceautomatically providing Mauchly’s Test for Sphericity and both theGreenhouse-Geisser and Hyunh-Feldt corrections in the event that thesphericity assumption is violated. Most importantly, however, theoutputs of these two functions agree when we look at the f-statisticsfor all main-effects and interactions when sphericity is assumed.
Manipulation checks are usually done at the end of the procedure to be sure that the effect of the manipulation lasted throughout the entire procedure and to avoid calling unnecessary attention to the manipulation. Manipulation checks become especially important when the manipulation of the independent variable turns out to have no effect on the dependent variable. Imagine, for example, that you exposed participants to happy or sad movie music—intending to put them in happy or sad moods—but you found that this had no effect on the number of happy or sad childhood events they recalled. This could be because being in a happy or sad mood has no effect on memories for childhood events.
(Multiple Crossed Factors)
Time of day (day vs. night) is represented by different locations on the x-axis, and cell phone use (no vs. yes) is represented by different-colored bars. It would also be possible to represent cell phone use on the x-axis and time of day as different-colored bars. The choice comes down to which way seems to communicate the results most clearly. The bottom panel of Figure 5.3 shows the results of a 4 x 2 design in which one of the variables is quantitative.
Factorial Designs
But in many ways, the complex design of this experiment undertaken by Schnall and her colleagues is more typical of research in psychology. Fortunately, we have already covered the basic elements of such designs in previous chapters. In this chapter, we look closely at how and why researchers combine these basic elements into more complex designs. We start with complex experiments—considering first the inclusion of multiple dependent variables and then the inclusion of multiple independent variables. There is an interaction effect (or just “interaction”) when the effect of one independent variable depends on the level of another.
In the bottom panel, independent variable “B” again has an effect at both levels of independent variable “A,” but the effects are in opposite directions. Figure 8.4 shows the strongest form of this kind of interaction, called a crossover interaction. One example of a crossover interaction comes from a study by Kathy Gilliland on the effect of caffeine on the verbal test scores of introverts and extraverts (Gilliland, 1980)[2].
If they were low in private body consciousness, then whether the room was clean or messy did not matter. There is an interaction effect (or just “interaction”) when the effect of one independent variable depends on the level of another. A 2×2 factorial design is a type of experimental design that allows researchers to understand the effects of two independent variables (each with two levels) on a single dependent variable. An approach to including multiple independent variables in an experiment where each level of one independent variable is combined with each level of the others to produce all possible combinations. Often a researcher wants to know how an independent variable affects several distinct dependent variables. As another example, researcher Susan Knasko was interested in how different odors affect people’s behavior [Kna92].
Also notice that each number in the notation represents one factor, one independent variable. So by looking at how many numbers are in the notation, you can determine how many independent variables there are in the experiment. 2 x 2, 3 x 3, and 2 x 3 designs all have two numbers in the notation and therefore all have two independent variables. The numerical value of each of the numbers represents the number of levels of each independent variable. A 2 means that the independent variable has two levels, a 3 means that the independent variable has three levels, a 4 means it has four levels, etc. To illustrate, a 3 x 3 design has two independent variables, each with three levels, while a 2 x 2 x 2 design has three independent variables, each with two levels.
No comments:
Post a Comment